The Importance of Utilizing a Medical Dataset for Machine Learning
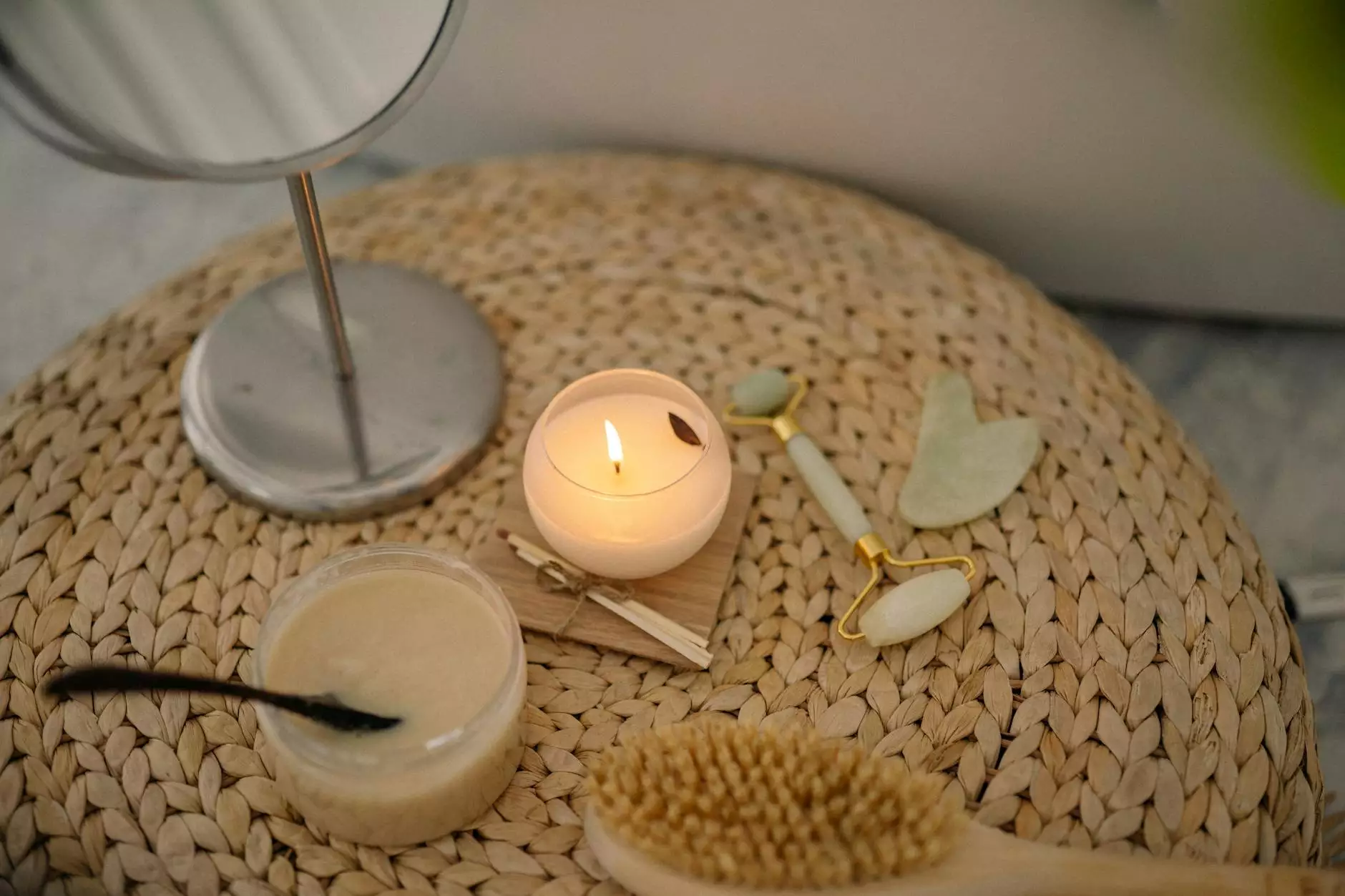
In today's rapidly advancing technological environment, the integration of machine learning (ML) into the healthcare sector has revolutionized how medical professionals approach diagnosis, treatment, and patient care. At the heart of this transformation lies the access to comprehensive and high-quality medical datasets for machine learning. These datasets form the backbone of algorithms that drive predictive analytics, support clinical decision-making, and ultimately enhance patient outcomes. This article delves deep into the significance of medical datasets in machine learning, highlighting their applications, benefits, and the future they herald for the healthcare industry.
What is a Medical Dataset?
A medical dataset is a structured collection of health-related data that can be used for analysis and decision-making in the medical field. Such datasets can include a wide range of information, like:
- Patient demographics (age, gender, ethnicity, etc.)
- Medical history (past illnesses, surgeries, family history)
- Clinical data (diagnoses, treatments, medications)
- Laboratory results (blood tests, imaging results)
- Patient outcomes (recovery rates, complications)
The richness and variety of this information make medical datasets invaluable for training machine learning models that aim to improve healthcare delivery. Utilizing these datasets enables healthcare professionals to glean insights that would otherwise remain obscure.
The Role of Machine Learning in Healthcare
Machine learning, a subset of artificial intelligence, focuses on building systems that learn from data rather than relying on explicit programming. In healthcare, ML plays a pivotal role in numerous applications, including:
1. Predictive Analytics
Healthcare providers utilize machine learning to predict patient outcomes based on historical data. For example, algorithms can identify patients at risk of developing complications post-surgery or those who may be predisposed to chronic diseases.
2. Diagnostic Support
ML algorithms can analyze medical images, such as X-rays and MRIs, to identify abnormalities much faster than human radiologists. This not only speeds up the diagnostic process but increases accuracy, reducing the risk of human error.
3. Personalized Medicine
By analyzing genetic data alongside clinical records, machine learning can help in tailoring treatments to individual patients, thereby enhancing the effectiveness of care while minimizing side effects.
4. Drug Discovery and Development
Machine learning enables researchers to identify potential drug candidates more efficiently by analyzing vast datasets of chemical compounds and their biological impacts. This can dramatically reduce the time and cost associated with drug development.
Benefits of Using Medical Datasets for Machine Learning
The utilization of medical datasets for machine learning brings forth numerous advantages that enhance the quality of care provided by healthcare systems:
1. Enhanced Decision-Making
The most significant advantage of machine learning in healthcare is improved decision-making. With access to robust datasets, practitioners can make evidence-based decisions, leading to better patient outcomes.
2. Cost Reduction
Through improved efficiency, machine learning can reduce operational costs significantly. Predictive analytics allows healthcare providers to anticipate needs and streamline processes, minimizing waste and optimizing resource allocation.
3. Improved Patient Outcomes
By leveraging ML's capabilities in analyzing clinical data, healthcare professionals can deliver more accurate diagnoses and effective treatments, leading to improved recovery rates and patient satisfaction.
4. Continuous Learning
Machine learning models evolve as new data is fed into them, allowing for continuous improvement in predictions and recommendations. This adaptability is crucial in rapidly changing medical landscapes.
The Challenges in Utilizing Medical Datasets
While the advantages are substantial, there are noteworthy challenges associated with the use of medical datasets for machine learning:
1. Data Quality and Consistency
Medical datasets often contain incomplete or inconsistent data. For effective machine learning applications, ensuring data quality is paramount. Cleaning and preprocessing the data can be a time-consuming and complicated task.
2. Privacy Concerns
Healthcare data is sensitive and protected by regulations such as HIPAA (Health Insurance Portability and Accountability Act). Ensuring patient privacy while still utilizing their data for training models presents a significant challenge.
3. Bias in Data
Machine learning models can potentially perpetuate or amplify biases present in the datasets. It is crucial to ensure diverse and representative data collection to improve model fairness and reduce health disparities.
4. Interoperability
Different healthcare systems may use varied formats and standards for their data. Achieving interoperability between systems to facilitate comprehensive data analysis remains a hurdle within the industry.
Examples of Medical Datasets Used in Machine Learning
Several publicly available medical datasets for machine learning are currently being utilized by researchers and practitioners to develop advanced healthcare solutions:
1. MIMIC-III
The Medical Information Mart for Intensive Care (MIMIC-III) is a freely accessible critical care database that includes real-world data from tens of thousands of ICU admissions. This expansive dataset supports research in areas like predictive modeling for patient outcomes and clinical decision support systems.
2. The Cancer Genome Atlas (TCGA)
TCGA is a collaboration that has generated a comprehensive genomic data set for various cancers, allowing researchers to study cancer biology and develop targeted therapies through advanced machine learning techniques.
3. PhysioNet
PhysioNet provides free access to large collections of physiological and clinical data. Their datasets are commonly used for machine learning applications focused on cardiovascular studies and various health condition analyses.
4. UCI Machine Learning Repository
This repository includes numerous datasets for various domains, including several medical-related datasets perfect for research and machine learning model training.
Future Trends in Medical Datasets and Machine Learning
As we move further into the technological era, the importance of medical datasets for machine learning will only continue to rise. Here are some future trends to watch:
1. Increased Use of Real-Time Data
The incorporation of real-time data from wearable devices and IoT (Internet of Things) technologies is expected to grow. This data will enrich medical datasets and support proactive and personalized patient care.
2. Enhanced Data Privacy Measures
With growing concerns about data privacy, advancements in secure data sharing methods, like federated learning, will play a critical role in easing access to crucial datasets while protecting patient information.
3. Ethics and Regulation Development
The conversation surrounding the ethics of using medical datasets will advance, mandating a framework of regulations to ensure ethical use of data in machine learning applications.
4. Cross-Disciplinary Collaboration
As healthcare becomes increasingly data-driven, collaboration between healthcare professionals and data scientists will become essential for extracting maximum value from medical datasets.
Conclusion
In conclusion, the medical dataset for machine learning is more than just data; it represents a monumental shift in how healthcare is practiced and delivered. By leveraging these datasets effectively, we can expect significant improvements in patient care, operational efficiency, and overall healthcare outcomes. Though challenges exist, the push towards a data-centric approach to healthcare is paving the way for a brighter, healthier future. As stakeholders continue to invest in data quality, ethical considerations, and innovative applications, the potential of machine learning in transforming healthcare will be realized. Embracing this change is not just beneficial; it is essential for the future of medical practice.