Unlocking the Power of Image Annotation Tool for Machine Learning
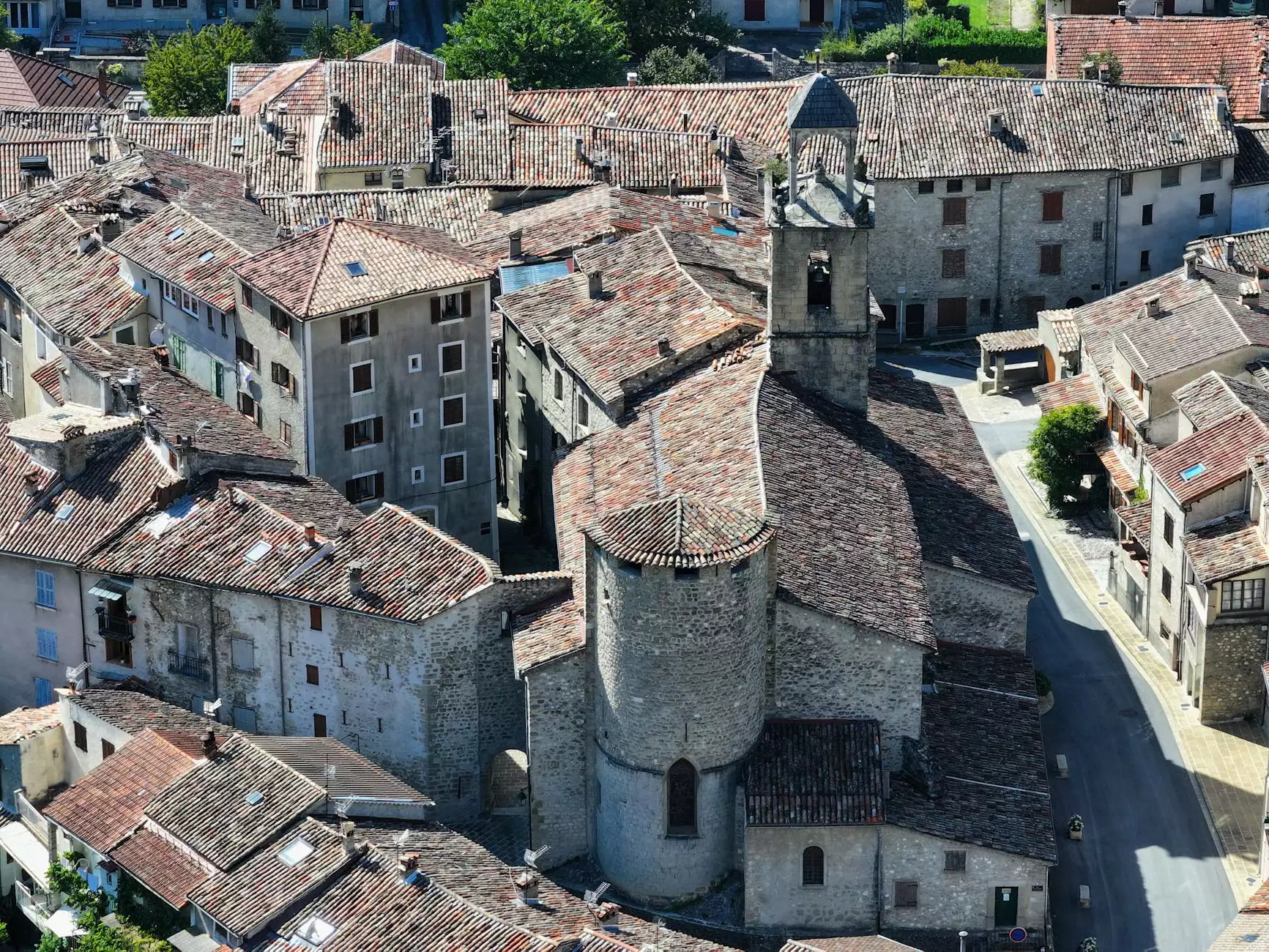
In the world of machine learning, data is king, and effective data annotation is crucial. As businesses increasingly turn to artificial intelligence (AI) and machine learning (ML) to drive innovation, the demand for high-quality, accurate data annotation tools has skyrocketed. Among these tools, the image annotation tool for machine learning stands out as a critical enabler of robust training datasets.
The Importance of Data Annotation in Machine Learning
Data annotation refers to the process of labeling data to make it understandable for machines. For machine learning algorithms to learn successfully, they require extensive datasets that have been labeled effectively. This is particularly true for computer vision tasks, where the ability to accurately annotate images is crucial. The image annotation tool for machine learning simplifies this complex process.
Types of Data Annotation
Data annotation can take various forms, including:
- Image Annotation: Adding labels or tags to images.
- Text Annotation: Categorizing textual data for natural language processing.
- Video Annotation: Tagging frames in videos for action recognition.
- Audio Annotation: Labeling sounds and voices for speech recognition.
Why Use an Image Annotation Tool?
Choosing the right image annotation tool for machine learning can significantly impact the efficiency and effectiveness of your ML projects. Below are some compelling reasons to invest in a high-quality annotation tool:
1. Improved Accuracy and Quality
Manual annotation can be prone to errors. A dedicated image annotation tool incorporates features that enhance accuracy. Automated suggestions and tools for ensuring consistency eliminate human error.
2. Time Efficiency
Using an image annotation tool greatly reduces the time spent on data preparation. High-powered tools allow for batch processing and streamlined workflows, enabling teams to focus on the analysis and development stages.
3. Scalability
As your dataset grows, so does the need for more annotation. Good image annotation tools can manage large datasets and enable collaborative workflows among team members, making scaling up projects much easier.
4. Versatility
Many tools cater to various annotation types, including bounding boxes, segmentation, and landmarking. This versatility means your annotation tool can adapt to different projects—whether it's for autonomous vehicles, facial recognition, or medical imaging.
Key Features of an Effective Image Annotation Tool
When exploring the best image annotation tools for machine learning, look for the following features:
1. User-Friendly Interface
A clean, intuitive interface enhances productivity. Effective visualization tools help annotators focus and reduce the likelihood of errors.
2. Customization and Flexibility
Your annotation tool should allow for customizable workflows to suit your project's specific needs. This flexibility will accommodate various use cases and methodologies, providing you with the necessary frameworks for your data.
3. Advanced Annotation Techniques
Features such as polygonal segmentation, 3D annotation, and point cloud annotation can add immense value, enabling you to tackle even the most complex datasets.
4. Integration Capabilities
To effectively manage your machine learning projects, your annotation tool should integrate seamlessly with other platforms and tools you utilize, such as data management systems and machine learning models.
How KeyLabs.ai Stands Out
Among the myriad of data annotation platforms, KeyLabs.ai emerges as a leader with its innovative approach to image annotation. By utilizing state-of-the-art technology and a robust platform, KeyLabs.ai offers a comprehensive data annotation tool that meets the diverse needs of businesses.
1. Quality Assurance Protocols
KeyLabs.ai employs rigorous quality assurance protocols to ensure that every piece of annotated data meets industry standards. This commitment to quality minimizes the risk of errors that could derail machine learning models.
2. Collaborative Environment
Collaboration is crucial in any ML project. KeyLabs.ai provides a platform that fosters collaboration among team members, allowing for real-time feedback and adjustments to ensure the highest quality annotations.
3. Comprehensive Training Resources
KeyLabs.ai recognizes that user education is paramount. With a wealth of training resources and guides, users can quickly learn how to maximize the functionalities of the image annotation tool for machine learning.
Implementing Image Annotation in Machine Learning Projects
To employ an image annotation tool effectively, follow these general steps:
- Define Your Requirements: Understand and outline what you need to annotate and the types of labels required.
- Select a Tool: Choose an annotation tool that offers the necessary features for your project.
- Train Annotators: Ensure that your team is adequately trained on how to use the tool and understand the annotation guidelines.
- Maintain Quality Control: Regularly review annotated data to ensure consistency and accuracy.
- Integrate with ML Models: Once annotation is complete, integrate the data into your machine learning workflows effectively.
Challenges in Image Annotation and How to Overcome Them
While data annotation is essential, the process is not without its challenges:
1. High Volume of Data
With the proliferation of data, managing and annotating large datasets can become overwhelming. To tackle this, employing a scalable annotation tool like KeyLabs.ai can help manage workload efficiently.
2. Maintaining Consistency
Different annotators may have varying interpretations of labels. Establishing a clear guideline and constant communication among team members is vital to maintaining consistency.
3. Budget Constraints
Investing in high-quality annotation tools can be costly. However, the return on investment in terms of the quality of the trained ML models and the resultant insights can be substantial.
The Future of Image Annotation in Machine Learning
As machine learning continues to evolve, so too will the image annotation tools. Emerging technologies such as automated annotation via AI, active learning, and federated learning will likely become integral components of future annotation efforts.
Embracing Automation
Automated annotation tools are expected to save significant time by quickly generating labels for vast datasets. However, human oversight will still be necessary to ensure these annotations meet quality standards.
Leveraging AI for Enhanced Annotation
By harnessing machine learning algorithms, future image annotation tools will become more sophisticated, providing features such as self-learning capabilities and predictive suggestions for labels, making the image annotation tool for machine learning even more indispensable.
Conclusion
The rise of artificial intelligence and machine learning creates an ever-growing need for superior data annotation tools. The image annotation tool for machine learning represents a vital component of this landscape, providing the essential services required to train effective ML models. KeyLabs.ai stands at the forefront of this technological advancement, offering innovative solutions that empower businesses to achieve their data annotation goals. By investing in high-quality image annotation tools and understanding the integral role they play, businesses can harness the full potential of their machine learning initiatives.
For more information on how to implement effective image annotation, visit KeyLabs.ai and discover comprehensive solutions tailored to your business needs.